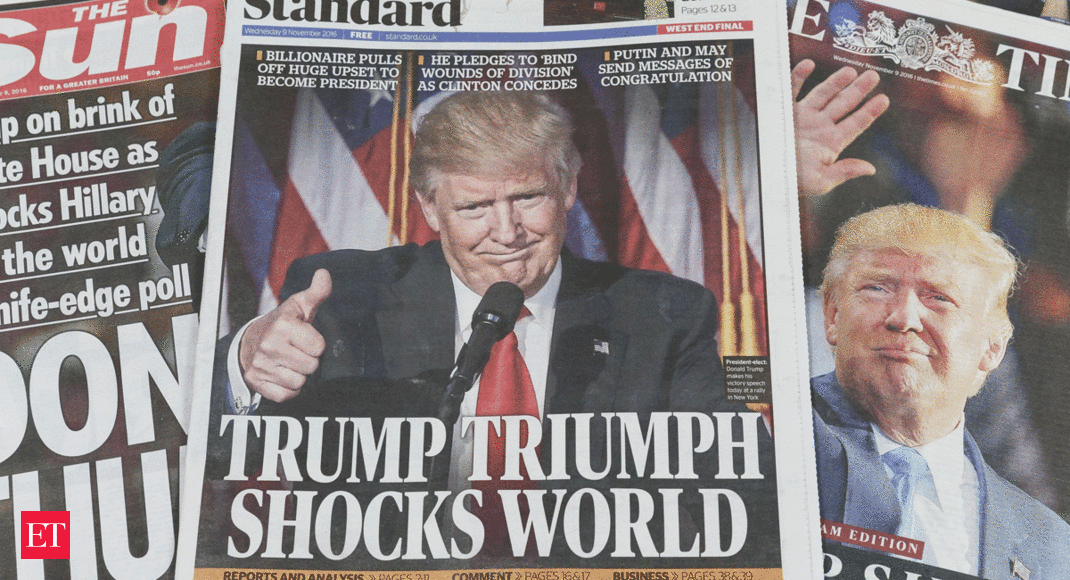
If we’re worried about getting drenched on the way to work, we take a look at what meteorologists’ computer models say about the weather. If the weather report tells us there’s an 80 percent chance of rain, we bring an umbrella.
With all the anxiety about Tuesday’s vote, it’s understandable that many of us look to statisticians’ election models to tell us what will happen. If they say your candidate has an 80 chance of winning, you feel reassured.
But after Donald Trump’s surprising victory in 2016 seemed to defy those models, there have been many questions about how much attention we should pay to electoral forecasting.
There’s a strong case for ignoring the predictions.
Why do we have models? Why can’t we just consider polling averages? Well, because presidents are not elected by a national vote total but by the electoral votes of each state, national polls do not give us the information we need. As two of the last five elections showed — in 2000 and 2016 — it’s possible to win the popular vote and lose the Electoral College. Models give us a way to process polls of various quality in 50 states to arrive at a forecast.
There are two broad ways to model an event: using “fundamentals” — mechanisms that can affect the event — and probabilities — measurements like polls. For elections, fundamentals would be historically informed lessons like, “a better economy favors incumbents.” With polls, there is no theory about why they are the way they are. We just use the numbers they produce.
Electoral forecast modelers run simulations of an election based on various inputs — including state and national polls, polling on issues and information about the economy and the national situation. If they ran, say, 1,000 different simulations with various permutations of those inputs, and if Joe Biden got 270 electoral votes in 800 of them, the forecast would be that Mr. Biden has an 80 percent chance of winning the election.
This is where weather and electoral forecasts start to differ. For weather, we have fundamentals — advanced science on how atmospheric dynamics work — and years of detailed, day-by-day, even hour-by-hour data from a vast number of observation stations. For elections, we simply do not have anything near that kind of knowledge or data. While we have some theories on what influences voters, we have no fine-grained understanding of why people vote the way they do, and what polling data we have is relatively sparse.
Consequently, most electoral forecasts that are updated daily — like those from FiveThirtyEight or The Economist — rely heavily on current polls and those of past elections, but also allow fundamentals to have some influence. Since many models use polls from the beginning of the modern primary era in 1972, there are a mere 12 examples of past presidential elections with dependable polling data. That means there’s only 12 chances to test assumptions and outcomes, though it’s unclear what in practice that would involve.
A thornier problem is that unlike weather events, presidential elections are not genuine ‘repeat’ events. Facebook didn’t play a major role in elections until probably 2012. Twitter, without which Mr. Trump thinks he might not have won, wasn’t even founded until 2006. How much does an election in 1972, conducted when a few broadcast channels dominated the public sphere, tell us about what might happen in 2020?
Interpreting electoral forecasts correctly is yet another challenge. If a candidate wins an election with 53 percent of the vote, that would be a decisive victory. If a probability model gives a candidate a 53 percent chance of winning, that means that if we ran simulations of the election 100 times, that candidate would win 53 times and the other 47 times — almost equal odds.
In its final forecast in 2016, FiveThirtyEight gave Hillary Clinton a 71.4 percent chance of victory. (The digit after the decimal providing an aura of faux precision, as if we could distinguish 71.4 percent from 71.5 percent.) All that figure really said was that Mrs. Clinton had a roughly one-in-three chance of losing, something that did not get across to most people who saw a big number. Most sites gave an even bigger number, with The New York Times predicting Mrs. Clinton had an 85 percent chance of winning on the day of the vote.
Since 2016, sites like FiveThirtyEight have gotten much better at presentation, focusing on odds and scenarios, and even explicitly urging people to remember that upset wins are possible. Still, the point of a forecast is to predict, and people may not be that likely to think “anything can happen” when they see what appear to be overwhelming odds in one direction.
One key problem in 2016 was the assumptions pollsters made when modeling the electorate — the people who would actually show up to vote. Pollsters were a little off in estimating the educational level of the electorate, especially in the Midwest. What’s more, people who settled on a preference late were a bit more prone to vote for Mr. Trump, and his supporters were a bit more likely to turnout than the models assumed. Even small shifts like that matter greatly; if it’s happening in one state, it’s probably happening in many similar states.
In 2020, it’s even harder to rely on polls or previous elections: On top of all the existing problems with surveys in an age of cellphones, push polls and mistrust, we’re in the middle of a pandemic. What do the unprecedented early voting numbers mean when polls don’t necessarily stop polling those who already voted? How do the early forecasts that run for many months before the election, and so are even more uncertain, affect those who vote early? Will the elderly, at great risk from the pandemic, avoid voting? How will voter suppression play out? Will Republicans end up flocking to the polls on Election Day? These are big unknowns that add great uncertainty to models, especially given the winner-takes-all setup in the Electoral College, where winning a state by as little as one-fourth of 1 percent can deliver all its electoral votes.
There’s an even more fundamental point to consider about election forecasts and how they differ from weather forecasting. If I read that there is a 20 percent chance of rain and do not take an umbrella, the odds of rain coming down don’t change. Electoral modeling, by contrast, actively affects the way people behave.
In 2016, for example, a letter from the F.B.I. director James Comey telling Congress he had reopened an investigation into Mrs. Clinton’s emails shook up the dynamics of the race with just days left in the campaign. Mr. Comey later acknowledged that his assumption that Mrs. Clinton was going to win was a factor in his decision to send the letter.
Similarly, did Facebook, battered by conservatives before the 2016 election, take a hands-off approach to the proliferation of misinformation on its platform, thinking that Mrs. Clinton’s odds were so favorable that such misinformation made little difference? Did the Obama administration hold off on making public all it knew about Russian meddling, thinking it was better to wait until after Mrs. Clinton’s assumed win, as has been reported?
Indeed, in one study, researchers found that being exposed to a forecasting prediction “increases certainty about an election’s outcome, confuses many, and decreases turnout.” Did many people think like Edward Snowden, who famously tweeted to millions of followers 18 days before Election Day, “There may never be a safer election in which to vote for a third option,” appending a New York Times forecast claiming that Hillary Clinton had a 93 percent chance of victory to his tweet? Did more Clinton voters stay home, thinking their vote wasn’t necessary? Did more people on the fence feel like casting what they thought would be a protest vote for Donald Trump? We’ll never know.
When probability models first came on the scene, I was hopeful that they would lessen the horse-race journalism that sometimes exaggerated the uncertainty (because what’s the thrill otherwise?) and the search for narrative turning points (Better than expected debate performance! It’s an underdog comeback!). I had hoped that we would instead get more substantive, policy-oriented coverage. Instead, modeling has been incorporated into the horse-race coverage.
And given all the uncertainty, misunderstanding and fragility of electoral forecasts, I’m not sure there is a meaningful difference between, say, 20 percent and 40 percent chances of winning. That’s another way of saying these forecasts aren’t that useful, and may even be harmful if people take them too seriously.
Instead of refreshing the page to update predictions, people should do the only thing that actually affects the outcome: vote, donate and organize. Everything else is within the margin of error.
"really" - Google News
November 01, 2020 at 11:49PM
https://ift.tt/2JstKWP
Trump's surprise 2016 win and why you can't really put your faith in election forecasts - Economic Times
"really" - Google News
https://ift.tt/3b3YJ3H
https://ift.tt/35qAk7d
Bagikan Berita Ini
0 Response to "Trump's surprise 2016 win and why you can't really put your faith in election forecasts - Economic Times"
Post a Comment